Evaluating Player effectiveness for Scouting and Recruitment through football datasets
- Vignesh Jayanth
- Feb 25, 2021
- 2 min read

The inspiration behind this study comes from effectively rating players to its closest proximate real-world scenario. Due to the unforthcoming nature of sharing data in the football world, teams sometimes use data from different sources to add context to the analysis of players. Football Manager Data has been used for scouting and recruitment by many teams over the past years.
Ole Gunnar Solskjaer, Manchester United’s head coach has openly admitted back in 2013 that he learned a lot about management of tactics from playing the Football Manager game (Hamilton, 2020).
Hence, this study uses data from a very detailed player-oriented attributes dataset that shows the overall ability of a player along with a variety of metrics deemed important for player scouting. The depth of player analysis available in the datasets allow for variety of analysis evaluating the player and the team to identify a suitable match between them.
The study will relate the analysis to the micro-level aspect of understanding player performance. A micro concept would be based on the idea of using a four-corner model to measure player development amongst professional footballers namely Physical, Technical, Psychological and Tactical. This holistic view of player analysis as suggested by (Simmons, 2004) is used by the national football governing body in England i.e. the Football Association (FA) to accelerate and evaluate player development from youth academies all the way to the first team in professional football. Third, the study will investigate different visualizations used by professional teams and novel visualizations to improve the speed of decision making by professional teams.
In summary, the study aims to make the following contributions:
1) Form a cluster analysis to identify best match for player transfer. The study will provide real world transfers that have been successful to identify the accuracy of the cluster analysis
2) Produce Data Analysis and Data Visualizations to show team and player strength thereby making an effective comparison between them

Using PCA, the data was fit and transformed into clusters (groups) of similar player profiles using their attributes
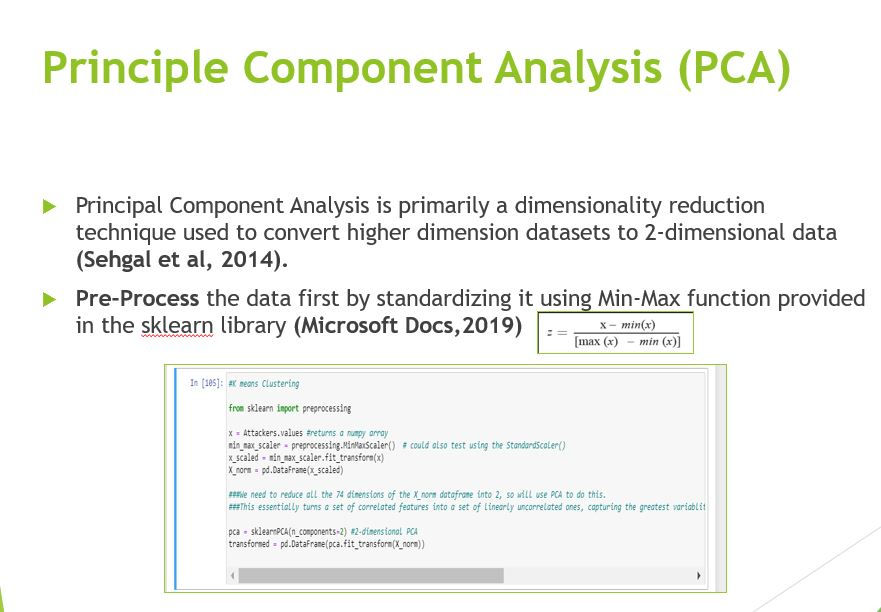
The Optimal number of clusters can be defined using the elbow method based on a point in the curve where variance in sum of squared errors decreases. In this study, the optimal number of clusters was 5.

The study produced player portfolios amongst which Philippe Coutinho (Liverpool) was in the same group as Frank Ribery (Bayern). As seen below, the study looked at real world transfers and a visual view of attributes of players.
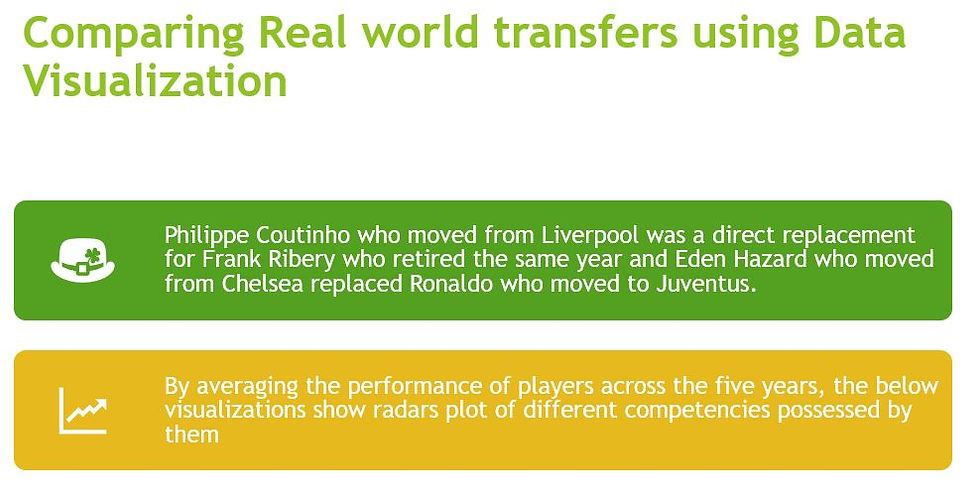
Philippe Coutinho <-> Frank Ribery
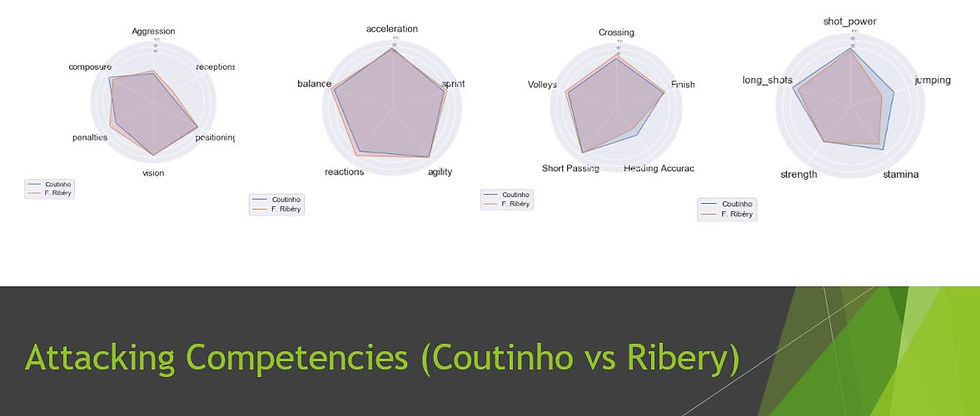
Eden Hazard<-> Cristiano Ronaldo

Kommentare